Transforming your digital future with NLP and NLU in data analysis
NLP vs NLU: What’s the Difference and Why Does it Matter? The Rasa Blog
He is a technology veteran with over a decade of experinece in product development. He is the co-captain of the ship, steering product strategy, development, and management at Scalenut. His goal is to build a platform that can be used by organizations of all sizes and domains across borders.
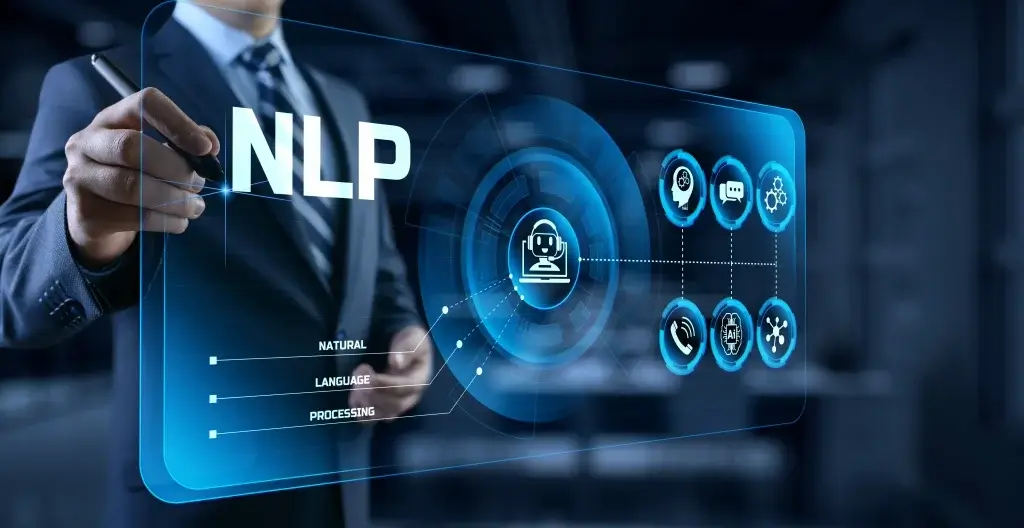
Since customers’ input is not standardized, chatbots need powerful NLU capabilities to understand customers. The procedure of determining mortgage rates is comparable to that of determining insurance risk. As demonstrated in the video below, mortgage chatbots can also gather, validate, and evaluate data. For instance, the address of the home a customer wants to cover has an impact on the underwriting process since it has a relationship with burglary risk. NLP-driven machines can automatically extract data from questionnaire forms, and risk can be calculated seamlessly.
Textual Analysis: Definition, Approaches and Examples
NLU helps computers understand the text they are given and its nuances, and NLG helps them produce useful output. Together, they form NLP, an artificially intelligent computing system that understands humans and the nitty-gritty of human language. Syntax analysis focuses on sentence structure to understand grammar and other aspects of an input text. The semantic analysis builds zeros in on the meaning of the input data in the given context. And sentiment analysis helps them understand the overall emotional quotient in relationship with the entities mentioned in the content.
5 Major Challenges in NLP and NLU – Analytics Insight
5 Major Challenges in NLP and NLU.
Posted: Sat, 16 Sep 2023 07:00:00 GMT [source]
Meanwhile, our teams have been working hard to introduce conversation summaries in CM.com’s Mobile Service Cloud. They say percentages don’t matter in life, but in marketing, they are everything. The customer journey, from acquisition to retention, is filled with potential incremental drop-offs at every touchpoint.
NLU vs NLP: What’s the Difference?
Deploying a rule-based chatbot can only help in handling a portion of the user traffic and answering FAQs. NLP (i.e. NLU and NLG) on the other hand, can provide an understanding of what the customers “say”. Without NLP, a chatbot cannot meaningfully differentiate between responses like “Hello” and “Goodbye”. Evolving from basic menu/button architecture and then keyword recognition, chatbots have now entered the domain of contextual conversation.
- These can then be analyzed by ML algorithms to find relations, dependencies, and context among various chunks.
- Both NLP& NLU have evolved from various disciplines like artificial intelligence, linguistics, and data science for easy understanding of the text.
- All these sentences have the same underlying question, which is to enquire about today’s weather forecast.
- It is quite possible that the same text has various meanings, or different words have the same meaning, or that the meaning changes with the context.
NLU is nothing but an understanding of the text given and classifying it into proper intents. Together with NLG, they will be able to easily help in dealing and interacting with human customers and carry out various other natural language-related operations in companies and businesses. However, when it comes to handling the requests of human customers, it becomes challenging. This is due to the fact that with so many customers from all over the world, there is also a diverse range of languages. At this point, there comes the requirement of something called ‘natural language’ in the world of artificial intelligence.
When are machines intelligent?
The ability to process human language is of course essential in things like Conversational AI, and another good real-life example of a use of NLP is a chatbot. Botpress described NLP as “what makes a chatbot feel human” — and they’re right in saying this, because the ability to comprehend human language allows chatbots to communicate with us in a way that we can understand. Natural Language Understanding provides machines with the capabilities to understand and interpret human language in a way that goes beyond surface-level processing. It is designed to extract meaning, intent, and context from text or speech, allowing machines to comprehend contextual and emotional touch and intelligently respond to human communication. While NLP converts the raw data into structured data for its processing, NLU enables the computers to understand the actual intent of structured data. NLP is capable of processing simple sentences,NLP cannot process the real intent or the actual meaning of complex sentences.
- For example, it is difficult to directly compare studies given the range of different methods, techniques, and outcomes.
- However, when it comes to understanding human language, technology still isn’t at the point where it can give us all the answers.
- NLP breaks down the language into small and understable chunks that are possible for machines to understand.
Natural Language Understanding(NLU) is an area of artificial intelligence to process input data provided by the user in natural language say text data or speech data. It is a way that enables interaction between a computer and a human in a way like humans do using natural languages like English, French, Hindi etc. If a developer wants to build a simple chatbot that produces a series of programmed responses, they could use NLP along with a few machine learning techniques. However, if a developer wants to build an intelligent contextual assistant capable of having sophisticated natural-sounding conversations with users, they would need NLU. NLU is the component that allows the contextual assistant to understand the intent of each utterance by a user.
Introduction to NLP, NLU, and NLG
Read more about https://www.metadialog.com/ here.